Introduction: Colorado Riverflow and Climate Change
Climate change stands as one of the most pressing environmental challenges of our time, with widespread consequences that impact ecosystems, economies, and communities alike. Water resources are among the most critical aspects affected, facing increasing strain as global temperatures rise and precipitation patterns shift. In Colorado, where river systems are the lifeblood of both urban and rural communities, these changes are particularly significant. Approximately 83% of Colorado’s drinking water comes from river systems, which also provide vital water resources to several downstream states, including Arizona, Nevada, and California. The impacts of climate change are already evident in Colorado’s river systems, as streamflows have decreased by an estimated 3% to 19% since 2000 compared to the historical average from 1951 to 2000. This decline is driven by diminished snowpack, earlier snowmelt, and altered precipitation patterns, and it is expected to worsen, further straining water supplies.
Reduced snowpack, earlier snowmelt, and changing precipitation patterns have a direct impact on water availability in the region. These issues are compounded by rising global temperatures, which intensify the severity and frequency of drought conditions. As these climate-related factors continue to worsen, they will have cascading effects on agriculture, industry, and residential water use. The consequences extend far beyond Colorado, affecting millions of people and industries across the southwestern United States that rely on the state’s rivers for drinking water, irrigation, and hydropower generation.
The Colorado River, in particular, is a vital water source for the southwestern United States, serving over 40 million people and supporting agriculture, industry, and recreation. However, the river’s flow is highly variable, influenced by a combination of environmental factors such as temperature fluctuations, precipitation levels, and snowpack accumulation in the mountainous headwaters. The complex relationship among these factors makes predicting future riverflow a significant challenge, but one that is essential to address in the context of climate change. This project seeks to tackle this challenge by using advanced machine learning techniques to predict future riverflow in the Colorado River Basin. By leveraging historical riverflow data, snowpack measurements, and meteorological records—including precipitation and temperature data—this project will provide a comprehensive understanding of the factors influencing riverflow. Additionally, two Representative Concentration Pathway (RCP) scenarios will be incorporated: a moderate scenario and a severe scenario. These scenarios reflect potential climate change outcomes and allow for a more nuanced prediction of future conditions.
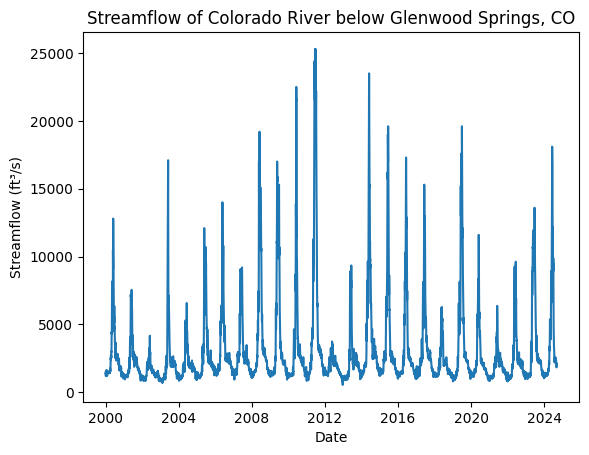
The primary objective of this project is to develop a predictive model capable of accurately forecasting riverflow in the Colorado River Basin. Such a model would serve as an invaluable tool for water managers tasked with making critical decisions about water allocation, drought mitigation, and long-term resource planning. By integrating climate projections with historical data, the model aims to provide actionable insights to help mitigate water shortages and ensure equitable distribution among stakeholders. Although extensive research has explored the impacts of climate change on water resources in Colorado, there remains a gap in the application of machine learning specifically for riverflow prediction in the Colorado River Basin. This project aims to fill that gap by analyzing the complex interactions between snowpack, snowmelt, precipitation, and temperature and their combined effects on riverflow, water supply, and agriculture.
The outcomes of this research could have far-reaching implications, not only for Colorado but also for the broader southwestern United States, where water scarcity is an increasingly urgent concern. As water resources become more constrained due to climate change, the importance of accurate and timely predictions will continue to grow. A reliable predictive model can inform strategies for sustainable water management, support policymakers in making evidence-based decisions, and help communities adapt to the challenges of a changing climate. This project represents a significant step toward safeguarding water supplies for the millions of people, industries, and ecosystems that depend on the Colorado River.
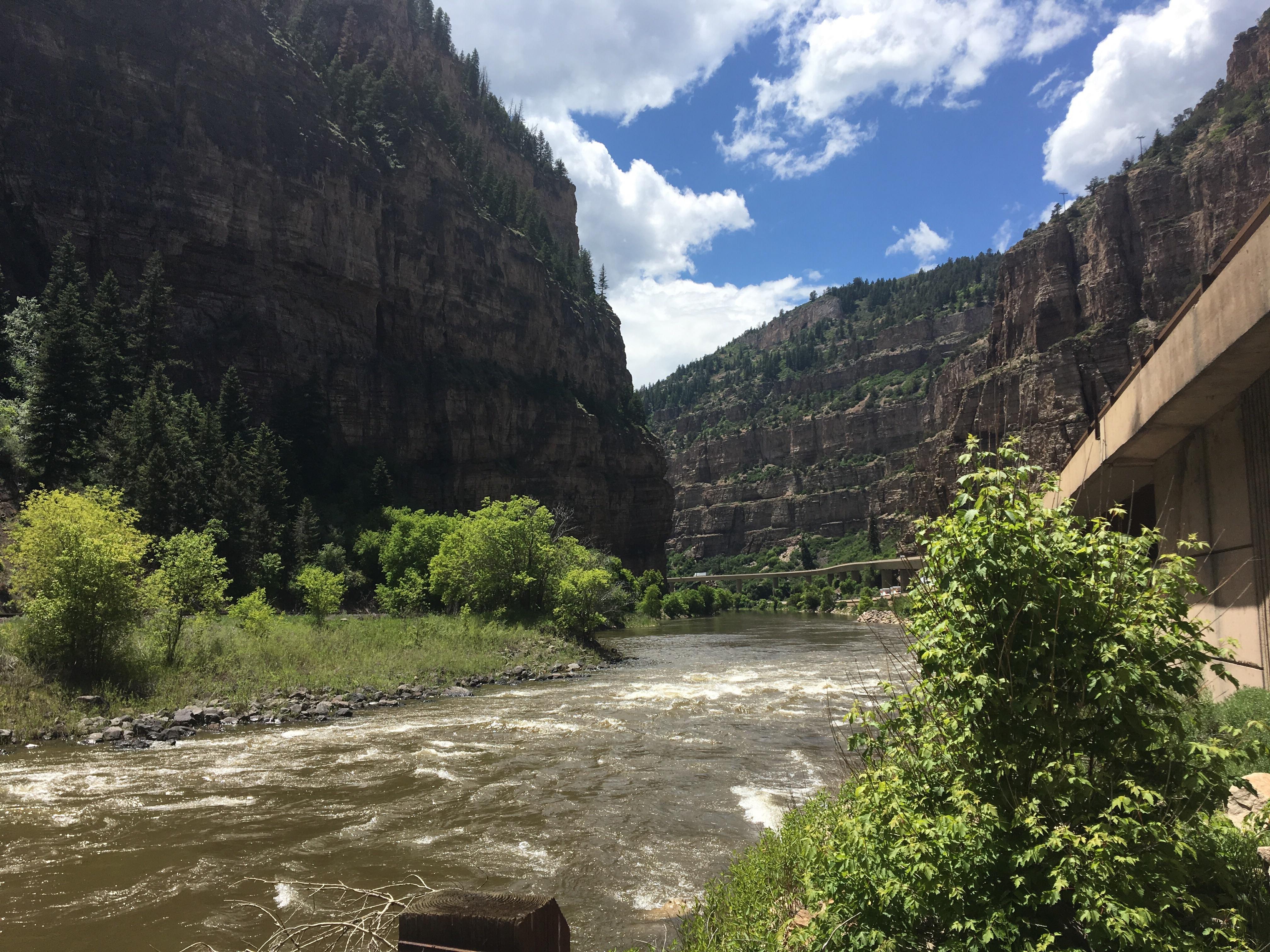
Key Questions
References
1. Bolinger, R.A., J.J. Lukas, R.S. Schumacher, and P.E. Goble, 2024: Climate Change in Colorado, 3rd edition. Colorado State University, https://doi.org/10.25675/10217/237323.
2. Barnett, T. P., Malone, R., Pennell, W., Stammer, D., Semtner, B., & Washington, W. (2004). The effects of climate change on water resources in the west: Introduction and overview. Climatic Change, 62(1-3), 1-11.
3. Udall, B., & Overpeck, J. (2017). The twenty-first century Colorado River hot drought and implications for the future. Water Resources Research, 53(3), 2404-2418.